Scientists’ ambitious attempt to make artificial intelligence thoughtful and creative.
The mere creation of artificial alternatives to natural intellect or, at least, of its separate aspects, allows us to understand the operation of our brain. In a recent past we could not have predicted the possibility of detecting voice or hand writing by the machines. However, the rocketing pace of a progress in IT industry has proven these tasks perfectly manageable. This technical evolution of artificial intelligence (AI) helps to justify the boundaries of human brain in a rather precise way, making the brain research more productive.
Owing to the effective system of modern innovations the idea that machines are incapable of replacing humans in any jobs becomes less attractive. Nowadays, scientists are aware of how to teach AI new skills. For example, two weeks ago American programmers informed about their know-how: the algorithms of an AI generate images in most popular art styles whilst also creating new art in an improvised manner. The first artworks have already been displaced to the wide audience, which could not distinguish between the art produced by machines and that of the real artists.
The idea of making machines to generate art is not new. One of the few existing systems of automatic art generation by AI was proposed by German researchers two years ago. Their artificial neurone network was capable of synthesising images from two independent sources: one source responded to the plot of the painting, another source to the art style. Speaking clearly, this system was capable of turning simple pictures and sketches into the art canvas preformed in styles of the world known artists.
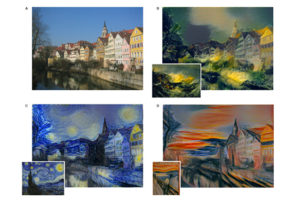
In 2016, Alex Shampandar, British developer, created another programme which could produce images in specific artistic styles. This programme was based on enveloping neurone network. The first step is to upload the picture with a style you need to turn any photo in. This enveloping neurone network scans the style of any particular artist and applies it to a customised picture.
Over a time period, the scientists took over responsibility to create the system that could be able to produce its own artistic vision without reproducing existing well known styles. In an overview to the research paper the scientists refer to the theory of comparative motivation of anglo-canadian psychologist Berlyle Deniel Elyse. Psychologist stated that psychophysical conception of excitement plays major role in exploration of aesthetic phenomenon. It is clear that we are more prone to appreciate novelty. Having the example of Pavlov’s dogs in our mind, we can argue that habituation leads to reduction of excitement and the following loss of interest in an artwork. On the other hand, we could quote the work of Martindayle Collin (USA): ‘The evolution and the end of an art era is a Hegelian tragedy’. Thus, with a reference to Hegel we might also add that the novelty of art has its limits- as far as it reaches certain degree of its unpredictability, it becomes misunderstood and, eventually, it dies.
After getting familiarised with the work of these researchers, the American programmers concluded that the main purpose of an artist is to create something new, but not something over futuristic. It was this particular action that the programmers wanted from their creation. The name of this newly created system is Creative Adversarial Network (CAN). It helps to generate all the existing art styles and create new artworks in unusual yet adaptive to viewer ways.
Speaking precisely, this algorithm is based on neuro network GAN ( Generative adversarial networks). It consists of two networks: the first is called generator, which processes data and suggests the second option (discriminator). The purpose of the latter is to define the source of data whilst the generator tries to be unpredictable. Maintaining the balance between the networks is an obstacle: if one becomes better than other then the algorithm will never match.
The so called “ Art- agent CAN” is built with the same principals. The discriminator has an access to the panel with different images and can distinguish art styles such as rococo and cubism. The purpose of the generator is to form the painting in a specific art style which is different from the existing styles. It also important for the discriminator to identify this style as a ‘new art’. The idea of the mechanism is explained: ‘maximizing deviation from established styles and maximizing deviation from art distribution. The developers were exercising the network, using the paintings from publicly accessible data base “Wiki Art 3”, which hosts 81449 paintings and 1191 artists starting from the 15th century.
The works of AI were showcased to art appreciators and general visitors of art galleries. The excitement of developers came unpredictably when they realised that the app did work as appreciators could not notice the difference. In fact, they misleadingly gave more value to the art works produced by AI compared to the works created by real artists.
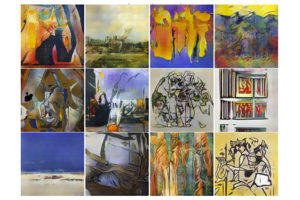
On the one hand, critics may say that this type of activity may have a limited objective. It is clear that no other artist existed in any century would have had such a cynical approach to the mystery of art as the developers of this system did. On the other hand, the mystery of art consists of the truth that every creator is unique and has its’ own vision. Nowadays, we readily accept the diversity of original thinking. In this case, what would be the reason not to accept the brain made with cables and integral schemes into our company? All in all, compared to the vast majority of ambiguous artists of all times, this brain, at least, does not fool itself.